Objectives of the Product
The FIPC has been specifically designed to address the growing onboard data bottleneck of EO satellite missions from a complete end-to-end systems perspective.
This is achieved primarily through a state-of-the-art Applications Development Framework which enables a software defined onboard data processing pipeline. Onboard data processing increases overall satellite bandwidth and data timeliness through direct data reduction and increased onboard intelligence. The objective is to provide users with actionable information much faster than traditional means and in turn stimulate new remote sensing applications which benefit from greater efficiency in the collection and processing chain. The framework not only enables the use of SSTL processing IP but also custom and third-party IP.
Direct data reduction techniques include data calibration, compression and image thumbnailing. Onboard intelligence includes image classification, segmentation and object detection; these enable high level information extraction for new data products and autonomy.
Additionally, the bottleneck is also addressed through a new, intelligent, high throughput data downlink system which uses variable modulation schemes and coding rates to utilise excess positive margin to increase the overall downlink throughput by up to 100%.
Customers and their Needs
The Earth Observation (EO) sector is undergoing a paradigm shift where technology advancements & new innovative payload designs are producing data with increasing dimensionality, volume and rates. In this new “Big Data” era a prominent challenge surrounds efficient and timely information delivery to end-users. In recent years, advances in satellite downlink technologies alone have not been sufficient to offset the increase in EO payload data volumes, resulting in the formation of an onboard data bottleneck.
This data bottleneck has a range of negative impacts to the customers. Principally it causes an increased delay between data capture and delivery. This delay has a wide range of knock-on impacts up and down the complete value chain, whereby data end-users can see the value of information decreased where required for time sensitive applications. Satellite operators can see increased delays in operations tasking and decision making. Satellite manufacturers need to address increased power, storage and complexity requirements of the satellite platform itself.
The bottleneck therefore can also have knock on impacts to higher level customers’ needs such as a negative impact on the customers overall business case due to increased missions costs. Costs are often increased by a need for greater ground station infrastructure or passes, increased satellite CapEx cost incurred to provide the required data volumes, resolutions or revisits.
Eliminating the onboard data bottleneck and time delay is a clear and urgent EO industry-wide opportunity, which, if successfully addressed, can provide a significant competitive advantage and stimulate new, time-sensitive or data heavy applications.
Targeted customer/users countries
SSTL primarily addresses the small satellite market; the FIPC will be included as part of SSTL’s small satellite mission offerings. The FIPC provides a significant advantage across SSTL’s mission offerings from the low cost CARBONITE series to SSTLs High Performance mission propositions, such as “Precision” and “Wide Swath”. Where previously the data bottleneck presented a significant challenge, with the FIPC customers are able to download larger volumes of data without cost prohibitive ground segment solutions. By decreasing the cost of the ground network needed to support the spacecraft, commercial business cases will become much more attractive to a wider range of customers.
Craft Prospect Limited (CPL) and their onboard Autonomy-Enabling Components (AEC) toolbox will be promoted as part of a staged product offering. Within the small satellite market, CPL will partner with SSTL to promote software AECs to enable and augment onboard data processing for SSTL smallsat missions.
CPL will offer three main tiers of products within the nanosatellite and spinout markets: the first tier will comprise the software AECs as standalone or packaged applications which can be deployed on third-party hardware or used in ground applications. The second tier will comprise dedicated hardware offerings, comprising the AECs and additional data processing functions optimised and deployed on low-power NewSpace components such as FPGAs and VPUs. The third tier will take the form of a full Autonomous Mission offering.
The University of Surrey are actively researching “big data” problems found in the context of the wider EO industry. One such avenue of research applicable to the FIPC product is the innovative use of autoencoder algorithms. Such algorithms can be deployed onboard the FIPC to extract contextualized information from EO data far quicker than traditional supervised learning approaches. The University of Surrey will demonstrate their novel algorithm developments and the new machine learning enabled customer use-cases.
Product description
The FIPC product consists of the space hardware required for; payload interfacing, data capture, data storage, real-time and offline onboard data processing and also the satellite RF downlink. However, the FIPC is more than just space hardware; the software defined onboard pipeline & applications development framework are critical components which enable end-user tailored & high performance functionality.
Overall, using a number of innovative techniques, the product is designed to maximise the achievable satellite throughput towards increased timeliness and volumes of useful payload data delivered to EO data end-users, thereby increasing value for money to the customer.
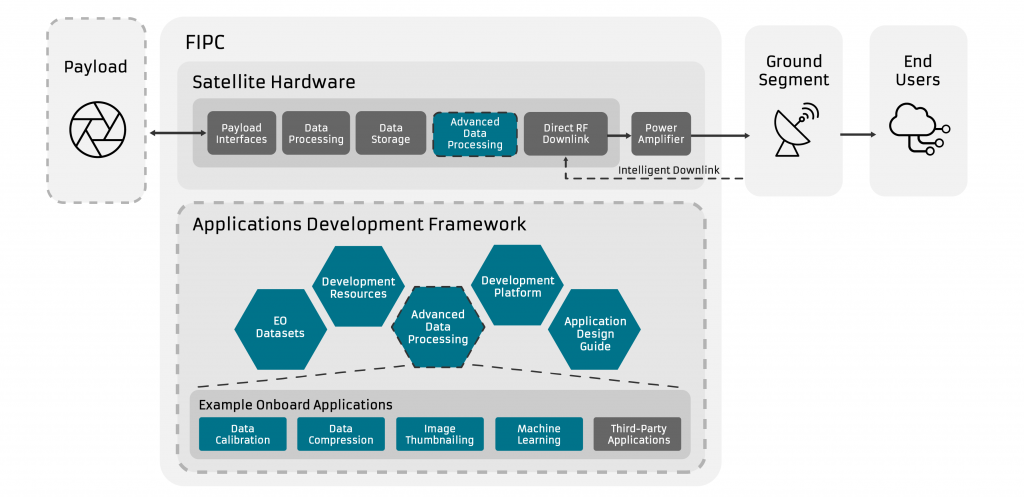
Added Value
EO data customers are increasingly demanding faster, cheaper, higher quality and greater volumes of data – this is a key challenge for EO satellites which is primarily limited by the achievable downlink throughput of the satellite. The FIPC leverages several different technological advances to increase the capabilities of the space segment and these are used to addresses customer demands from several different angles.
Firstly, an adaptive high data throughput downlink sub-system is provided to maximize the raw data transmission rate of the satellite.
Secondly, a number of new and novel approaches to onboard data processing are implemented at the front end. These can be used to reduce the volume of data needing to be sent via the downlink. Thus overall the product increases the volume, quality and timeliness of payload data to customers, providing better value for money.
Onboard data processing offerings include IP designed by SSTL, CPL and the University of Surrey.
Thirdly, The FIPC Applications Development Framework will allow customers and other third-party developers to deploy their own custom onboard data processing IP onboard an SSTL satellite allowing for highly optimised solution of EO data end-users.
In addition, the product is scalable across the range of SSTL satellite platform classes from 10s of kg to 100s of kg, whereby the FIPC offers utility across many types of remote sensing mission.
Current Status
The activity was kicked off in 2021.