Objectives of the Product
Extracting intelligence from Synthetic Aperture Radar (SAR) imagery is challenging and time-consuming. ATR4PAZ is an AI-driven toolbox designed for PAZ SAR satellite imagery, overcoming these hurdles. This toolbox swiftly analyses high-resolution SAR imagery, providing valuable information about objects of interest within minutes. Rigorous testing, involving real and simulated SAR imagery, ensures the accuracy and viability of this novel approach, combining cutting-edge AI models with SAR data.
ATR4PAZ addresses the limitations of traditional interpretation processes, enabling SAR-based Intelligence, Surveillance, and Reconnaissance (IMINT) to reach optical imagery standards. The successful implementation of ATR4PAZ not only meets current challenges but also opens avenues for scalable product development. This approach can evolve to detect and classify new types of objects of interest, marking a significant advancement in SAR-based IMINT capabilities. ATR4PAZ represents an efficient solution for rapid and accurate intelligence extraction from satellite imagery, with potential for broader applications in the future.
Customers and their Needs
In general, the ATR4PAZ product targets governmental customers working in intelligence and security that employ satellite imagery such as MoDs, intelligence agencies, police, customs, etc.
The main identified needs they have in relation to the use of SAR satellite imagery are:
- Automation of EO information extraction process in order to support rapid decision-making and mapping.
- A tool that can be adapted for automatic detection and classification of different objects of interest found in SAR imagery.
- The tool can also to be extended to include new types of objects.
- The tool must be accurate and can be improved with the assistance of photointerpreters.
Currently, the spanish MoD follows this activity in order to first hand know the accuracy of the results.
Analytics companies (EO downstream) who generate IMINT for Security and Intelligence users (as contractors). They have similar needs to the governmental customers.
Targeted customer/users countries
- MoD/SATCEN, Spain
- NGA, USA
- Value-adder and analytics, USA
- NATO countries
- EU countries
Product description
The main goal of the application is the sucessful detection and classification of objects that can be of interest to govermental customers in the framework of intelligence and Security. The picture below describes the overall ATR4PAZ tool that can be directly operated by a customer or used in the provision of a service where the customer receives only IMINT reports, avoiding the need of dealing with complex SAR imagery.
The tool automatically proccess SAR PAZ imagery, generating reports with the results, which can be supervised by the operator. This supervision of results is key for retraining AI models.
Supported by a SAR simulator for training AI models, new objects of interest can be added in different scenarios, making ATR4PAZ expandable to cater for new IMINT customers.
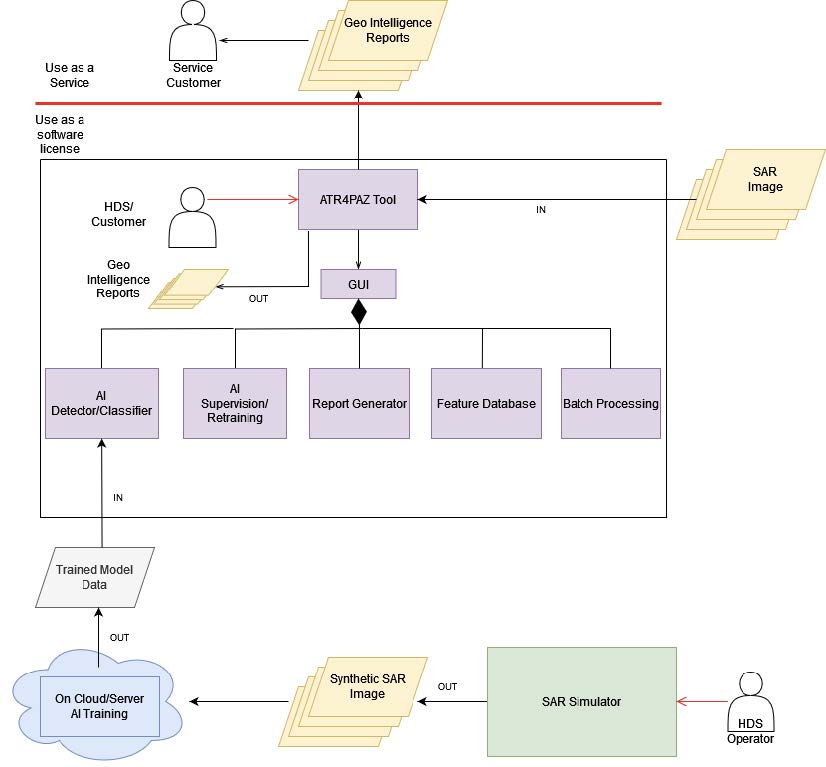
Added Value
The proposed de-risk activity aims to develop an operational tool for Automatic Target Recognition (ATR) using AI algorithms and PAZ satellite Synthetic Aperture Radar (SAR) images. Currently, AI techniques in ATR are limited due to the scarcity of large image datasets. The innovation lies in adapting a SAR simulator to generate realistic synthetic images from 3D object models, addressing the shortage of real satellite imagery. This approach offers significant advantages, including cost and time savings in acquiring training data.
Notably, there is a lack of successful and generalised ATR with SAR images in existing Earth Observation commercial software. While literature explores ATR with real images of specific objects, the proposed activity takes a pioneering step by combining synthetic and real SAR images to train AI algorithms. If successful, this approach would mark a significant milestone in SAR application development, demonstrating the feasibility of using simulated data in conjunction with real imagery for ATR training—an unprecedented achievement in the field.
Current Status
The project is ready for final review. To summarise, the main points are:
- PAZ SAR Image object labelling and AI model training. Different trade-off performances were carried out in order to find the best suitable mode for automatic detection/classification of objects. Results have exceeded the set detection/classification goals of the project, confirming that PAZ real data can suffice to train ATR software.
- SAR simulation/preprocessing. Simulation tools have been upgraded to improve the degree of realism of the SAR simulated imagery. ATR4PAZ has added the following new features:
- New SAR processor that enables the processing of long aperture SAR acquisitions.
- Object mask generation.
- Radiometric calibration, so simulations are compatible with PAZ SAR imagery.
- Comparison of SAR simulations with real PAZ imagery has revealed high degrees of similarity, although some additional improvements in the simulation tool are needed to make the simulation solution fully operational.